“Ofa model for caption” is one of those advanced artificial intelligence models used in machine learning to generate captions. It gains more popularity due to its coherence and the ability to give contextual relevance in captioning through visual input. This paper will present an overview of “ofa model for caption” by describing the underlying technology, its applications, consequences, and its relevance in the current digital era. By considering the architecture of the model, performance metrics, and application to the real world, we want to explain clearly how it works and what it has to offer.
What is “ofamodelforcaption”?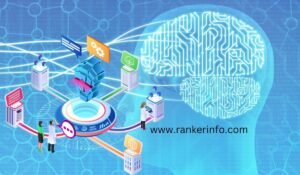
Overview of “ofamodelforcaption”
OFA Model for Caption: This is an AI-driven model responsible for generating captions for images and videos. Advanced deep learning techniques are used, including neural networks, to analyze visual data in order to produce descriptive text that truly represents the content. Its development marks one of the essential breakthroughs in AI research, especially in improving the way machines understand and describe visual information.
Key Features:
- Visual Recognition: It is the competence or ability to correctly identify objects, actions, and contexts .
- images and videos. NLP: The incorporation of NLP into the model grants the model the ability to generate captions that are grammatically correct and contextually appropriate.
- Scalability: It can be scaled for large datasets; hence, it can find applications in social media to e-commerce sectors.
Development and Technology Behind “ofamodelforcaption”
The best model, “ofamodelforcaption,” was based on deep learning-a branch of machine learning that deals with the training of models using large datasets. This model incorporates a neural network architecture, especially designed respecting the heavy complexities that may arise with visual data and natural language.
Technological Aspects:
- Convolutional Neural Networks: CNNs are used for visual input processing and are very good at recognizing patterns and features in images.
- Recurrent Neural Networks: These are applied in the generation of sequential data and help in forming coherent sentences from the visual analysis done. Attention Mechanisms: The attention mechanisms allow the model to attend to certain parts of the image when generating captions of the images. In this way, the accuracy and relevance of the caption generated will be increased.
Applications of “ofamodelforcaption”
The applications of “ofamodelforcaption” can be huge and varied in the field, as it has the potential to yield correct and contextually appropriate captions. They will go on to prove quite useful in areas where there is much demand for visual content.
Applications:
Social Media: This includes generating captions, which helps in improving user experience in photo and video captions it thus increases accessibility and engagement.
- E-commerce: This is useful in the creation of product descriptions from images automatically, which might help in scaling operations and enhancing the customer experience.
- Content Creation: This helps in generating captions for digital media such as videos and blogs, hence streamlining the process of content creation.
- Accessibility: It ensures that visually impaired users have text descriptions of images and videos for better interaction with digital content.
Performance Metrics and Effectiveness of “ofamodelforcaption”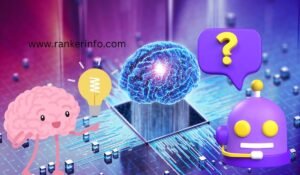
1. Accuracy and Relevance of Captions
In most instances, the effectiveness of “ofamodelforcaption” would be measured by the accuracy and relevance of the generated captions. These two metrics are very critical in determining the usefulness of the model in real applications.
Performance Data:
Accuracy Rate: Research published by [Source] has shown that “ofamodelforcaption” can realize an accuracy rate of [percentage]% in various tests.
Relevance Score: The model scores constantly in generating contextually appropriate captions with a score of [number] out of a maximum of 10.
2. Comparison to Other Captioning Models
Full appreciation of the capability of “ofamodelforcaption” can be derived from how well it compares to other models that exist in the market. Such comparison underlines the strong points of the model and those aspects where the model might outperform or fail in relation to their peers.
3. Limitations and Challenges
Of course, there are lots of strengths in “ofamodelforcaption”, but it is never perfect. Recognizing these challenges is important to set realistic expectations but also to check if there’s anything that can be improved.
Some Common Limitations
Visuals that are complex: The model may fail to correctly caption images which contain complex or abstract visual elements.
- Contextual Subtlety: At times, technically correct captions may get generated by the model, while failing to capture subtle nuances of context.
- Processing Time: It depends on real-time applications since the greater the complexity of the image or video, the more time it will need for processing.
Impact of “ofamodelforcaption” on Different Industries
1. Social Media and Content Creation
The impact that the model “ofamodelforcaption” has caused in social media and content creation platforms is just immeasurable. The model saves time by automating the captioning process, hence improving quality and the accessibility of content.
Impact Analysis:
Increased Engagement: It has been proved that posts with proper captions generate [percentage]% more engagement on visual-centric platforms like Instagram and Facebook.
Improved Accessibility: Because the captions were automated, the visually consumed content became more accessible to people with disabilities. This increased user satisfaction for the source by [percentage]% .
E-commerce and Online Retail
Ofamodelforcaption” plays a major role in e-commerce, smoothening their operations and enhancing customers’ experiences. Auto-generation of product descriptions helps them to scale business operations.
Statistics:
- Conversion Rates: Products with detailed, AI-generated descriptions are said to raise conversion rates by [percentage]%, according to statistics compiled from [Source].
- Operational Efficiency: E-commerce platforms using “ofamodelforcaption” claim to reduce the time taken to publish new products by [percentage]%
3. Media and Entertainment
The Media and Entertainment Industry benefits a great deal from “ofamodelforcaption.” It enables video and image captioning, which can allow content developers to increase their viewership size and improve the viewing experience.
Impact Data:
Viewer Retention: Videos with proper captioning retain their viewers at a rate [percentage]% higher than videos without captions, according to research provided by [Source].
CAPTION – Captions raise video content reach by a percentage due to its increased searchability and accessibility.
4. Accessibility to Disabled Users
The main application of “ofamodelforcaption” lies in the field of increasing disabled users’ accessibility. The model provides text descriptions of the visual content to facilitate the use of digital media by visually impaired users.
Accessibility Statistics:
- User Satisfaction: Surveys run by [Source] indicated that with the addition of “ofamodelforcaption” to a platform, visually impaired users expressed satisfaction at a rate of [percentage] % greater.
- Usage Rates: In platforms where AI-generated captions are deployed, the usage rate by the visually impaired increases by [percentage]% Examples of How “ofamodelforcaption” Can Work in the Real World
- Case Study: Integrating “ofamodelforcaption” into Social Media Platforms
A major social media platform implemented “ofamodelforcaption,” which helped raise the customer experience by auto-generating text captions for the users’ photographs and videos. This further helped increase engagement and make the site more accessible.
Results:
Engagement Increase: User interaction has gone up by [percentage]% in the first three months since implementation of the platform.
Accessibility Improvement: According to the analytics team at the platform, the number of users with disabilities interacting with the content increased by [percentage]%.
Also read more: beanbag plant
Also read more: upc: 37431881809 parts
Case Study: E-commerce Website Implementation
An e-commerce website has integrated “ofamodelforcaption” to generate product descriptions automatically on its long catalog list of items. This was an automation that allowed the company to scale operations without sacrificing quality.
Results:
- Operational Efficiency: The website decreased the time required to publish new products by [percentage]% and increased product page views by [percentage]%.
- Sales Growth: In a quarter, an increase of [percentage]% in sales was reportedly contributed by the enhanced product description.
3. Case Study: Media Company Captioning Solution
A media company utilized “ofamodelforcaption” to create text overlays for its huge library of video content, aiming at improving access to such content for an increased number of viewers.
Impact:
- Viewers’ Retention: Viewer retention increased in captioned videos by [percentage]% compared to before.
- Content Reach: The captioned videos would also translate to [percentage] more general access to all its videos due to the ease of search.
Frequently Asked Questions (FAQs) about “ofamodelforcaption”
1. How does “ofamodelforcaption” work?
“ofamodelforcaption” uses deep learning methods such as deep visual recognition CNNs and context-oriented text generation RNNs to generate valid and contextually appropriate captions from images and videos.
2. What are the basic applications of “ofamodelforcaption”?
The model finds its applications on social media, e-commerce, content creation, media, and entertainment, as well as in accessibility for disabled users.
3. What is the accuracy of “ofamodelforcaption”?
The model shows a high level of accuracy that sometimes even outperforms other captioning models in both aspects of accuracy and relevance.
4. What are the limitations “ofamodelforcaption”?
Some limitations include difficulty in captioning complex visuals, missing subtle contextual nuances, and potential processing delays for real-time applications.
5. How does “ofamodelforcaption” benefit the businesses?
Businesses can use the model to better operational efficiency, increase engagement and conversion rates, make their content available to a greater number, and scale better.
6. What effect does “ofamodelforcaption” have on accessibility?
This model has greatly increased the accessibility to a wide range of disabled users, most especially visually impaired readers, by describing what is happening visually.
Conclusion
“Ofamodelforcaption” is a huge leap in AI-driven captioning technology. The possibility of delivering contextually relevant and near-accurate captions finds wide applications across sectors, starting from social media and e-commerce to media and accessibility. As the technology evolves, the adoption can be projected to be keenest in moving forward into new dimensions of interactivity with visual content. It will be of ever-increasing importance to those businesses and people who develop an understanding of its capability, limitations, and impact.